kompowiec
freetard
- 2 582
- 2 649
https://money.cnn.com/2016/09/06/technology/weapons-of-math-destruction/index.html
Wealth: America's other racial divide
Math is racist: How data is driving inequality
By Aimee Rawlins September 6, 2016: 5:24 PM ET
It's no surprise that inequality in the U.S. is on the rise. But what you might not know is that math is partly to blame.
In a new book, "Weapons of Math Destruction," Cathy O'Neil details all the ways that math is essentially being used for evil (my word, not hers).
From targeted advertising and insurance to education and policing, O'Neil looks at how algorithms and big data are targeting the poor, reinforcing racism and amplifying inequality.
These "WMDs," as she calls them, have three key features: They are opaque, scalable and unfair.
Denied a job because of a personality test? Too bad -- the algorithm said you wouldn't be a good fit. Charged a higher rate for a loan? Well, people in your zip code tend to be riskier borrowers. Received a harsher prison sentence? Here's the thing: Your friends and family have criminal records too, so you're likely to be a repeat offender. (Spoiler: The people on the receiving end of these messages don't actually get an explanation.)
The models O'Neil writes about all use proxies for what they're actually trying to measure. The police analyze zip codes to deploy officers, employers use credit scores to gauge responsibility, payday lenders assess grammar to determine credit worthiness. But zip codes are also a stand-in for race, credit scores for wealth, and poor grammar for immigrants.
Cathy O'Neil
O'Neil, who has a PhD in mathematics from Harvard, has done stints in academia, at a hedge fund during the financial crisis and as a data scientist at a startup. It was there -- in conjunction with work she was doing with Occupy Wall Street -- that she become disillusioned by how people were using data.
"I worried about the separation between technical models and real people, and about the moral repercussions of that separation," O'Neill writes.
She started blogging -- at mathbabe.org -- about her frustrations, which eventually turned into "Weapons of Math Destruction."
One of the book's most compelling sections is on "recidivism models." For years, criminal sentencing was inconsistent and biased against minorities. So some states started using recidivism models to guide sentencing. These take into account things like prior convictions, where you live, drug and alcohol use, previous police encounters, and criminal records of friends and family.
These scores are then used to determine sentencing.
"This is unjust," O'Neil writes. "Indeed, if a prosecutor attempted to tar a defendant by mentioning his brother's criminal record or the high crime rate in his neighborhood, a decent defense attorney would roar, 'Objection, Your Honor!'"
But in this case, the person is unlikely to know the mix of factors that influenced his or her sentencing -- and has absolutely no recourse to contest them.
Or consider the fact that nearly half of U.S. employers ask potential hires for their credit report, equating a good credit score with responsibility or trustworthiness.
This "creates a dangerous poverty cycle," O'Neil writes. "If you can't get a job because of your credit record, that record will likely get worse, making it even harder to work
Wealth: America's other racial divide
Math is racist: How data is driving inequality
By Aimee Rawlins September 6, 2016: 5:24 PM ET
It's no surprise that inequality in the U.S. is on the rise. But what you might not know is that math is partly to blame.
In a new book, "Weapons of Math Destruction," Cathy O'Neil details all the ways that math is essentially being used for evil (my word, not hers).
From targeted advertising and insurance to education and policing, O'Neil looks at how algorithms and big data are targeting the poor, reinforcing racism and amplifying inequality.
These "WMDs," as she calls them, have three key features: They are opaque, scalable and unfair.
Denied a job because of a personality test? Too bad -- the algorithm said you wouldn't be a good fit. Charged a higher rate for a loan? Well, people in your zip code tend to be riskier borrowers. Received a harsher prison sentence? Here's the thing: Your friends and family have criminal records too, so you're likely to be a repeat offender. (Spoiler: The people on the receiving end of these messages don't actually get an explanation.)
The models O'Neil writes about all use proxies for what they're actually trying to measure. The police analyze zip codes to deploy officers, employers use credit scores to gauge responsibility, payday lenders assess grammar to determine credit worthiness. But zip codes are also a stand-in for race, credit scores for wealth, and poor grammar for immigrants.
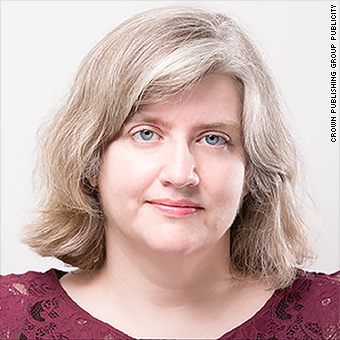
Cathy O'Neil
O'Neil, who has a PhD in mathematics from Harvard, has done stints in academia, at a hedge fund during the financial crisis and as a data scientist at a startup. It was there -- in conjunction with work she was doing with Occupy Wall Street -- that she become disillusioned by how people were using data.
"I worried about the separation between technical models and real people, and about the moral repercussions of that separation," O'Neill writes.
She started blogging -- at mathbabe.org -- about her frustrations, which eventually turned into "Weapons of Math Destruction."
One of the book's most compelling sections is on "recidivism models." For years, criminal sentencing was inconsistent and biased against minorities. So some states started using recidivism models to guide sentencing. These take into account things like prior convictions, where you live, drug and alcohol use, previous police encounters, and criminal records of friends and family.
These scores are then used to determine sentencing.
"This is unjust," O'Neil writes. "Indeed, if a prosecutor attempted to tar a defendant by mentioning his brother's criminal record or the high crime rate in his neighborhood, a decent defense attorney would roar, 'Objection, Your Honor!'"
But in this case, the person is unlikely to know the mix of factors that influenced his or her sentencing -- and has absolutely no recourse to contest them.
Or consider the fact that nearly half of U.S. employers ask potential hires for their credit report, equating a good credit score with responsibility or trustworthiness.
This "creates a dangerous poverty cycle," O'Neil writes. "If you can't get a job because of your credit record, that record will likely get worse, making it even harder to work